Stable Diffusion is a deep learning model that can generate detailed images from a short written description.
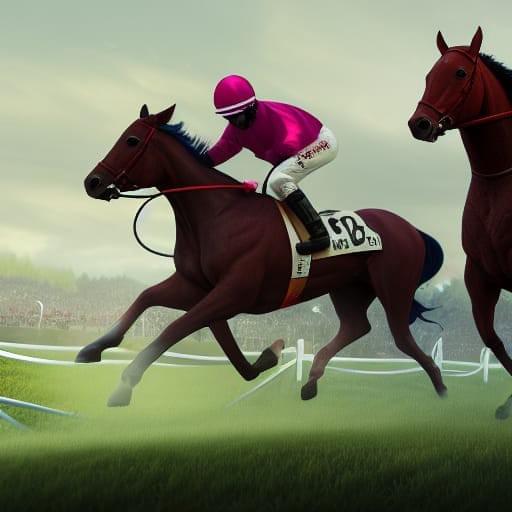
Deep learning is a subset of machine learning, which “gives machines an enhanced ability to find — and amplify– even the smallest of patterns [within data].”
However, some of the images generated by stable diffusion are stranger than fiction.
When asked to make a horse, the model will create around 20–25% of horse art with 5 legs.
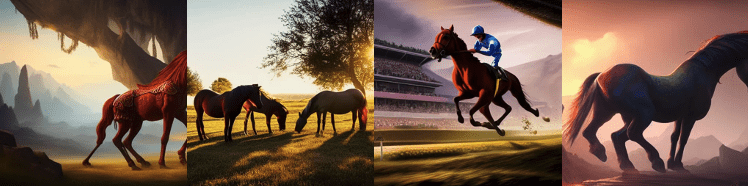
Gentle creatures with an extra leg.
Why?
Stable Diffusion occasionally creates 5-legged horses due to the data used to train the model. The training data includes a diverse amount of horse photos, which is confusing for the AI to determine the number of legs a horse actually has.
Hol’ up. Let’s back it up a little.
How does AI work?
Artificial intelligence learns from experience, which is in the form of data. Models are inferred functions that imitate patterns within the datasets the model was trained on. François Chollet lovingly refers to them as “big curves.”
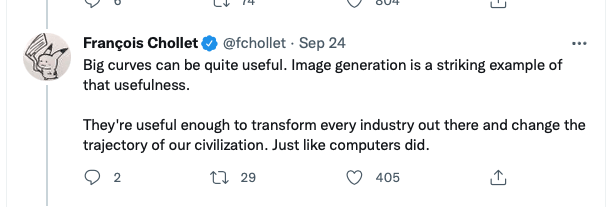
However, the human mind is not a big curve. A human can see 20,000 images of horses and understand that a horse has 4 legs. But stable diffusion cannot.
Under the hood: Stable Diffusion
You may be thinking: if AI learns from the data it was trained on, there must be some 5-legged horses in the datasets! You’re on the right track, but no cigar.
Stable Diffusion is trained on 20,000 images of horses. Many of these photos include occlusions, or when an object is hidden from view.
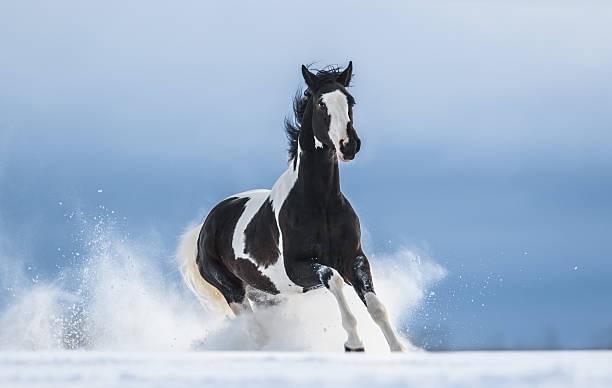
Snow flurries obscure the legs of a majestic stallion.
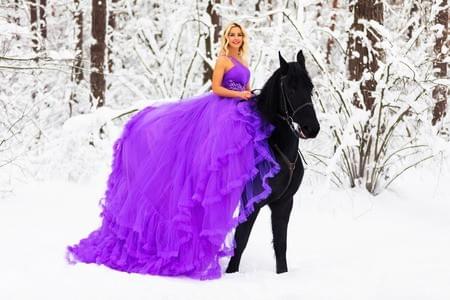
A fabulous dress blocks much of this black horse from view.
Another type of data that obscures leg count for AI are images of tails closely aligned with the legs of a horse.
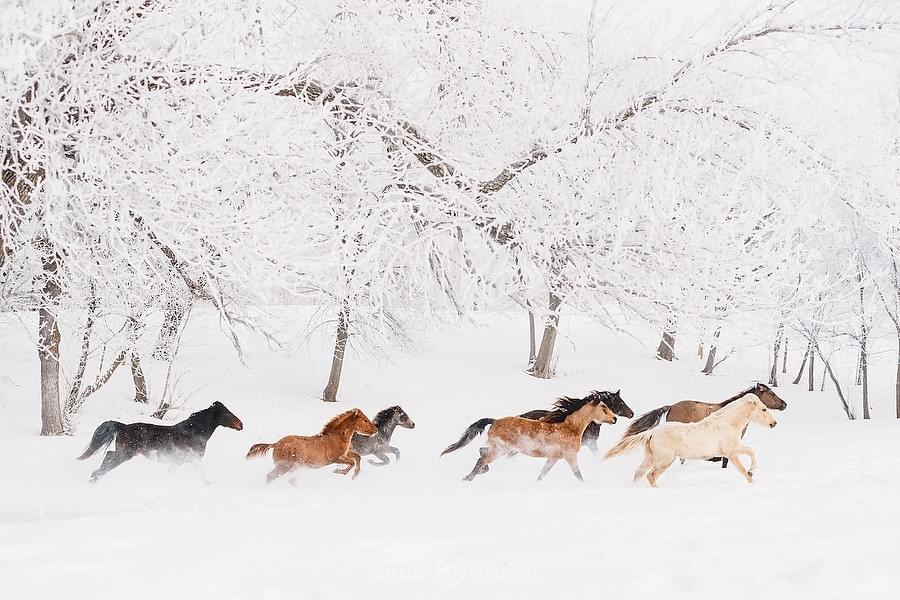
These horse legs and tails are similarly shaped.
The aesthetic of the image, such as horses in movement and artistic angles can cause confusion for the AI since those training examples are irregular and unique.
Overall, training data is the cause for challenges in AI to distinguish the number of legs a horse actually has, resulting in some strange-looking horses.
But bias in AI due to training data doesn’t end there.
Unethical AI behavior
In 2014, Amazon decided to shut down its experimental AI recruiting tool after discovering it discriminated against women. The tool crawled the web for potential candidates and rated them from 1 to 5 stars.
However, the algorithm learned to systematically downgrade women’s CVs for technical jobs, such as a software developer. It turns out that the tool was built using recruitment information from the last 10 years of hiring.
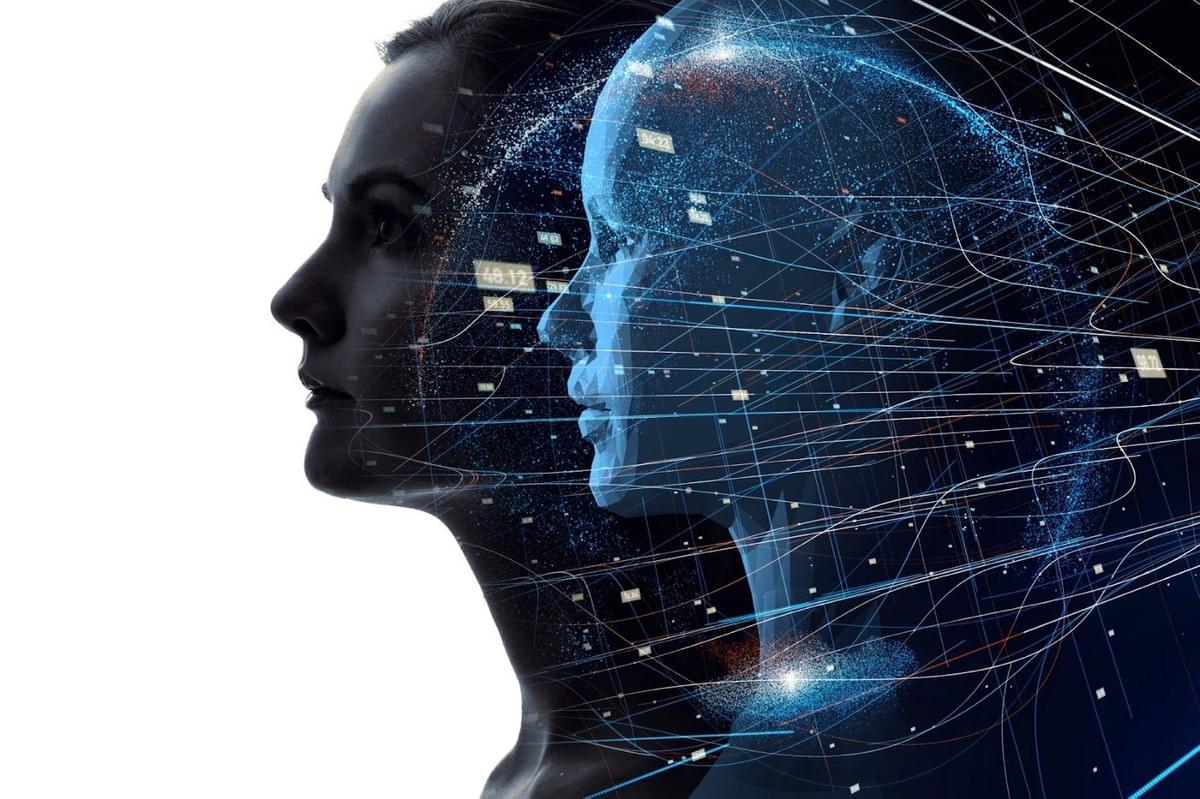
Historical data can include human-caused bias.
Wait a minute. Is AI more or less biased than humans? AI cannot reason through ethical decisions, like a human can. AI always takes the data it is given as true and morally permissible.
Machines also have difficulty understanding decision-making in our dynamic and messy world. “Context is everything. AI systems do not operate in isolation,” says Schwartz, principal investigator for AI bias at the National Institute of Standards and Technology (NIST). Good behavior in one context can change to bad in another.
In these moments, it’s good to recall François Chollet : human intelligence is a poor metaphor for what AI is actually doing. AI displays essentially none of the properties of human cognition, and in reverse, most of the useful properties of modern AI are not found in humans.
Overall, AI will make mistakes no matter what. But once we discover results we don’t want, we can incorporate that information into the training data and improve AI one step at a time.